The hippocampal circuits that store and recall spatial information are comprised of diverse cell types, each exhibiting distinct dynamics and complex patterns of synaptic connectivity. Thus, even highly specific experimental perturbations of a single component of these neuronal circuits can have counterintuitive effects on their internal dynamics and output. Computational modeling offers experimentalists a framework to integrate their knowledge, make explicit the assumptions of their conceptual models, and quantitatively predict how each element of a neuronal network is expected to respond to cell type- or projection-specific perturbations. In the Soltesz lab we build computational models in close collaboration with experimentalists, both in the lab, across Stanford, and at other institutions through a multi-site NIH BRAIN Initiative collaboration. Our large-scale network models of the hippocampus are continuously refined to incorporate newly obtained experimental constraints, and numerical simulations are carried out to test hypotheses, compare candidate biophysical and network mechanisms for memory storage and recall, and aide in the interpretation of physiological and behavioral experimental data. The ultimate goal of these efforts is to obtain a deep conceptual understanding of the cellular and network mechanisms that mediate “memory replay” events called sharp-wave ripples by simulating a large-scale model of the hippocampus that reproduces for the observed firing properties of all cell types during sharp-waves.
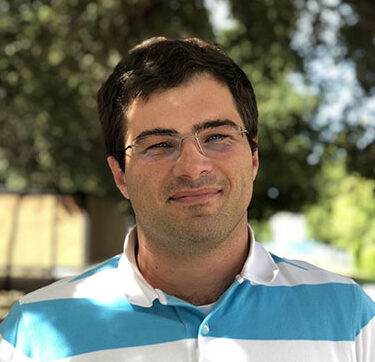
Research Engineer
Ivan Raikov
I hold undergraduate and master’s degree in Computer Science from the Georgia Institute of Technology, and a PhD in Biomedical Sciences from the University of Antwerp. I am studying information processing in the hippocampus by means of highly detailed and realistic computational simulation of neuronal networks at 1:1 scale. More broadly, I am interested in solving the enormous neuroinformatics challenges of computational neuroscience by developing sophisticated computational frameworks capable of expressing, organizing and managing the different types of data and algorithms associated with computational models of neural networks.
Email:iraikov@stanford.edu